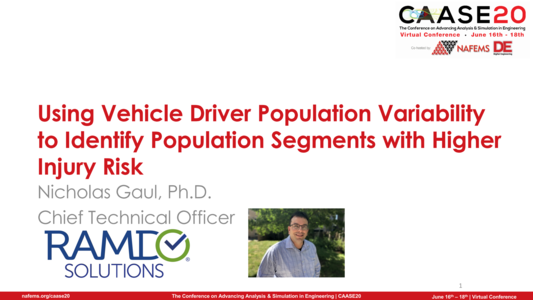
NAFEMS Americas and Digital Engineering (DE) teamed up (once again) to present CAASE, the (now Virtual) Conference on Advancing Analysis & Simulation in Engineering, on June 16-18, 2020!
CAASE20 brought together the leading visionaries, developers, and practitioners of CAE-related technologies in an open forum, unlike any other, to share experiences, discuss relevant trends, discover common themes, and explore future issues, including:
-What is the future for engineering analysis and simulation?
-Where will it lead us in the next decade?
-How can designers and engineers realize its full potential?
What are the business, technological, and human enablers that will take past successful developments to new levels in the next ten years?
Resource AbstractDesigning a vehicle restraint system is a complex and difficult problem to solve. One of the challenges is how to design a restraint system that works for all the various drivers. The variability in driver BMI, stature, age, and gender presents the engineer with a huge challenge to try to come up with a restraint system that can help reduce injury risk for all drivers. One solution is to design an adaptive restraint system that has different settings. The idea is that if all drivers would use the best setting for them, their injury risk would be reduced in the event of an accident; therefore, reducing the injury risk for all drivers. The new challenge then becomes how to segment the population into groups so that an adaptive restraint system can be designed to reduce the injury risk of each group. It will be shown how using the injury risk simulations together with uncertainty quantification and data science provides a method to segment the driver population into different groups that have higher injury risk. The variability of the driver BMI, stature, age, and gender is represented using distributions, which are obtained from data collected by the National Center for Health Statistics. These distributions are not known standard distribution types but are unique and even multimodal. This variability will be propagated using uncertainty quantification to obtain the variability in the predicted driver injury risk. The uncertainty quantification results will provide injury risk variability as distributions. Due to the complexity of the injury risk simulations and the nonstandard distributions representing the driver variability, the injury risk distributions are nonstandard distributions as well. Once the injury risk distributions are obtained it then becomes a data science problem to figure out how to use all this data to segment the driver population into groups that have high injury risk. It will be demonstrated how to use the driver variability distributions and injury risk distributions together in order to identify population segments with high injury risk.