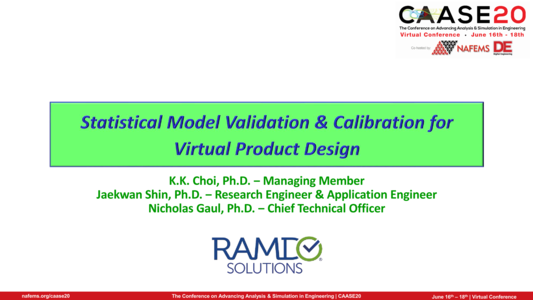
NAFEMS Americas and Digital Engineering (DE) teamed up (once again) to present CAASE, the (now Virtual) Conference on Advancing Analysis & Simulation in Engineering, on June 16-18, 2020!
CAASE20 brought together the leading visionaries, developers, and practitioners of CAE-related technologies in an open forum, unlike any other, to share experiences, discuss relevant trends, discover common themes, and explore future issues, including:
-What is the future for engineering analysis and simulation?
-Where will it lead us in the next decade?
-How can designers and engineers realize its full potential?
What are the business, technological, and human enablers that will take past successful developments to new levels in the next ten years?
Resource AbstractSTATISTICAL MODEL VALIDATION & CALIBRATION FOR VIRTUAL PRODUCT DESIGN
K.K. Choi, Jaekwan Shin and Nicholas Gaul
RAMDO Solutions, LLC, Iowa City, IA 52240, USA
kyung-choi@ramdosolutions.com, jaekwan-shin@ramdosolutions.com, nicholas-gaul@ramdosolutions.com
As industries are urged to make trade-offs between speed of product development, cost, quality and reliability, many are starting to develop Virtual Product Development (VPD) process. To develop an effective VPD process, it is critical that simulation models are statistically and quantitatively validated. However, there are a number of challenges to perform quantitative statistical simulation model validation and calibration in practical industrial applications: (1) limited numbers of input data for modeling input distributions, (2) possibly biased simulation models, (3) inaccurate surrogate models, and (4) limited numbers of output test data in the context of statistical and quantitative model calibration and validation. Numerous statistical model calibration and validation methods have been developed over two decades. However, many of them are too theoretical and complicated for practical use by industry engineers.
This development presents computational methods to generate simulation output distributions (uncertainty quantification) using the Dynamic Kriging (DKG) method, which automatically selects the best combination of basis and correlation functions for Kriging model from 54 possible combinations over the variance window for highly accurate and very efficient surrogate models. Using simulation model output distributions obtained from the DKG surrogate models and limited output test data, target output distributions, which provide good approximations of true output distributions, are obtained using Bayesian analysis.
These target output distributions are used to measure the biasness of simulation models for statistical model validation. In addition, an optimization based calibration (i.e., inverse problem) method is developed, by minimizing convex Hellinger distance (similarity) measure, for unknown input parameter distributions. Furthermore, the cumulative distribution function of the simulation model prediction error is proposed to provide (1) the most probable model prediction error and (2) model confidence level at a user-specified error, which are clear and easy for practicing engineers to use. Practical industrial application and analytical example for an internal combustion engine are used to demonstrate effectiveness of the developed statistical model validation & calibration methods.