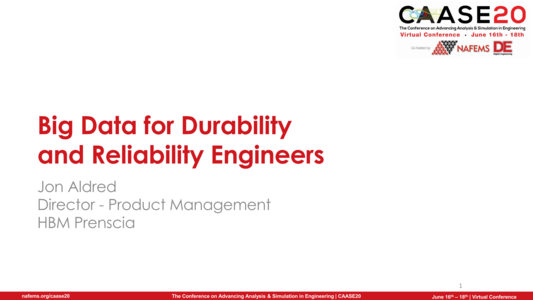
NAFEMS Americas and Digital Engineering (DE) teamed up (once again) to present CAASE, the (now Virtual) Conference on Advancing Analysis & Simulation in Engineering, on June 16-18, 2020!
CAASE20 brought together the leading visionaries, developers, and practitioners of CAE-related technologies in an open forum, unlike any other, to share experiences, discuss relevant trends, discover common themes, and explore future issues, including:
-What is the future for engineering analysis and simulation?
-Where will it lead us in the next decade?
-How can designers and engineers realize its full potential?
What are the business, technological, and human enablers that will take past successful developments to new levels in the next ten years?
Resource AbstractIntroduction:
The term big data is used in many ways and for many applications. The increased connectivity and availability of sensor data is driving new big data opportunities for both the design engineering and asset operations communities. However, the reality of unleashing this potential is perhaps more challenging than is often portrayed by major information technology vendors.
Product designers and OEMs are interested in understanding real product usage in order to enhance its functional design and make sure the new design is fit-for-purpose. For example, identifying the 95th percentile customer in terms of usage severity helps CAE and test engineers set representative design targets, thus reducing the risks of under or overdesign. Understanding usage information can also help to take important design decisions for example how usage differs around the world that may affect durability and warranty returns globally.
Operators responsible for a vehicle fleet or machines in a plant are typically looking to enhance product reliability. Historical data stored in a big data system can be coupled with analytics to build a mathematical model, which can be then used to predict upcoming failures based on day-to-day usage data. Predicting the remaining useful life of components can improve readiness and enable optimized maintenance activities by reducing unscheduled downtimes, which means more throughput and less costs. However, the adoption of such predictive analytics can be jeopardized by too many false positives. So, the model must be trained and made robust by incorporating as much historical data as possible. Machine Learning can be used to make a predictive model effective, but again, it requires large amounts of good, meaningful measured data and failure data in order to converge towards reliable predictions.
Common challenges of big data analysis:
Simply gathering and having access to large volumes of data is not helpful in giving insights to the health of an asset for instance, unless appropriate quality and analytics strategies are put in place. The first step is to ensure the data can be trusted. Experience shows that quality and cleanliness are more important than quantity in the world of big data. Another concern of analyzing very large volumes of data is the ability of applying known workflows to much larger datasets. In the past, engineers would conduct time-at-level histogram calculations on heavily instrumented test vehicles on proving grounds, but now they want to also perform the same types of time-at-level calculations on regular vehicles on public roads. Existing software tools are often not appropriate or provide a performance bottleneck. General open source tools for handling big data systems are necessary to access the large volumes of data. However, they offer relatively little mathematical functionality and are often insufficient for performing real-world engineering analysis on time series data.
Scaling up for real world applications:
One of the primary challenges is how to scale the data handling, data management and data analytics of measured sensor data to real world applications. This presentation will include an example of a framework and components for handling and processing condition based maintenance datasets from a fleet of vehicles. It demonstrates the scalability required to processing data from 20,000 vehicles, 40 channels per vehicle, 2 hours of usage per day for 3 years, generating 11 million data files and approximately 100TB of searchable time series data to enable timely decisions on vehicle performance, reliability and operations.