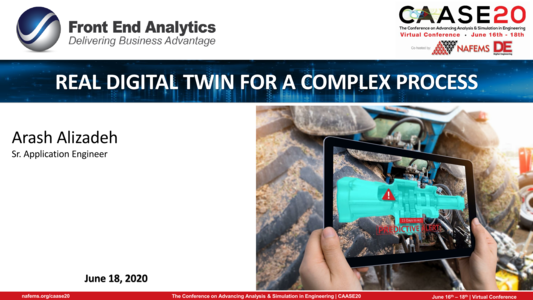
NAFEMS Americas and Digital Engineering (DE) teamed up (once again) to present CAASE, the (now Virtual) Conference on Advancing Analysis & Simulation in Engineering, on June 16-18, 2020!
CAASE20 brought together the leading visionaries, developers, and practitioners of CAE-related technologies in an open forum, unlike any other, to share experiences, discuss relevant trends, discover common themes, and explore future issues, including:
-What is the future for engineering analysis and simulation?
-Where will it lead us in the next decade?
-How can designers and engineers realize its full potential?
What are the business, technological, and human enablers that will take past successful developments to new levels in the next ten years?
Resource AbstractIn this paper we showcase the development of a fast running and highly predictive fatigue failure surrogate model based on data obtained from simulation runs of a complex mechanical system. The goals for the surrogate model were to create a Simulation Democratization tool for design exploration and a Digital Twin tool for field predictive maintenance and adaptive controls.
Catastrophic failure or sudden failures that are caused by damage accumulation are very difficult to predict using field data. However, in the design process, to ensure that parts meet operational life requirements, catastrophic failure analysis using simulation is routine. Often these simulations are complex and incorporate multiple physical phenomena interacting in complex and highly coupled ways. Given this, simulations are often time consuming, labor intensive, and require expensive software.
In the example being showcased, we faced the challenge of converting data from a small number of simulation runs for prediction of fatigue failure in an engine exhaust manifold, into a highly predictive surrogate model that could be used to predict real-time damage accumulation as expected failure point in field deployed products. Additionally, the same surrogate model could be used for design exploration. Thus, both operational and geometric variables were considered in the prediction of fatigue life and damage accumulation in the part.
To accomplish these goals, we used our in-house Physics Informed Machine Learning (PIML™) technology and methods. The PIML™ approach has better accuracy than the traditional statistical and machine learning methods and requires a fraction of the data required for training.
The outcome of this project was a physics informed PIML?? model that only used 17 simulation runs or experiments for training. The overall R² value for the PIML?? model was 0.97 & the average log error of the predicted life was 2% when compared to finite element simulation. Note that each finite element simulation took 3 weeks to run. Given this long run time and its expense, the use of conventional machine learning methods, which would have required thousands of simulation runs to create a low error surrogate for this problem, would have been impractical, if not impossible.
The implications of PIML™ to industrial applications, where predictive maintenance and predictive failure are cost and revenue drivers, are enormous. The conventional approach has relied, with minimal success, on conventional machine learning techniques to identify patterns in field data that may indicate the advent of a failure. However, damage accumulation and fatigue are seldom produce a discernable signal that is anomalous to prior operational data. Moreover, field failures take time, and catastrophic failures are seldom recorded in the field with enough accuracy and frequency to train a machine learning model. With PIML™ we open the opportunity to use Simulation as a tool for Digital Twin generation.