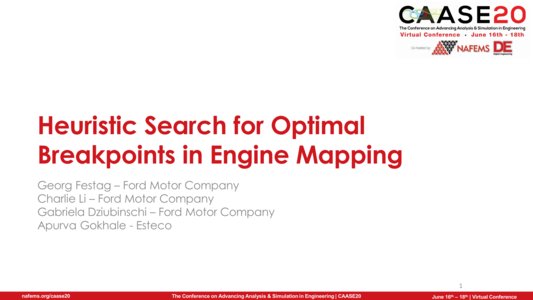
NAFEMS Americas and Digital Engineering (DE) teamed up (once again) to present CAASE, the (now Virtual) Conference on Advancing Analysis & Simulation in Engineering, on June 16-18, 2020!
CAASE20 brought together the leading visionaries, developers, and practitioners of CAE-related technologies in an open forum, unlike any other, to share experiences, discuss relevant trends, discover common themes, and explore future issues, including:
-What is the future for engineering analysis and simulation?
-Where will it lead us in the next decade?
-How can designers and engineers realize its full potential?
What are the business, technological, and human enablers that will take past successful developments to new levels in the next ten years?
Resource AbstractEngine mapping calibration deals with large amount of test data. When the calibrator generates an engine mapping table, the calibrator leverages his personal experience and trial and error method is applied. This approach slows down the engine development and validation. In addition, the trial and error method may create inaccurate engine mapping table, which may result in a waste of time and other resources. To improve the fidelity of maximum brake torque sparking timing tables, a robust process for the selection of optimal load break points of the timing tables is developed and validated. This robust process utilizes artificial intelligence to do a heuristic search of finite set of load break points. When the optimal set of load break points is found, the high-fidelity tables are generated for each operating condition.
The breakpoints should be valid and consistent for hundreds of driving conditions. Each condition may be drastically different when compared with others. To support the robust process an optimization strategy is developed. The process consists of the following steps: 1. Pre-processing (padding for missing test cases, filtering for noisy data and sorting) of engine test data at relevant operating conditions, 2. Apply optimization strategy to the cleaned data, 3. Run optimization of the load break points using heuristic algorithm, 4. Validation of the optimal load break point based on calculated error of maximum brake torque spark timing, 5. Generation of the high-fidelity engine mapping tables at desired operating conditions. Normally, the engine mapping table is a 2D table, the horizontal header represents the engine speeds while the vertical header represents the engine loads. The high-fidelity engine mapping tables require a value for each combination of engine load and speed. The test, however, cannot be run under certain combinations of engine load and speed due to test limitations and/or resource allocation. To generate appropriate engine mapping tables when the data is not collected for certain load and speed combinations, the padding is applied in the paper. To demonstrate the process, this paper presents a case study on the I4 engine.