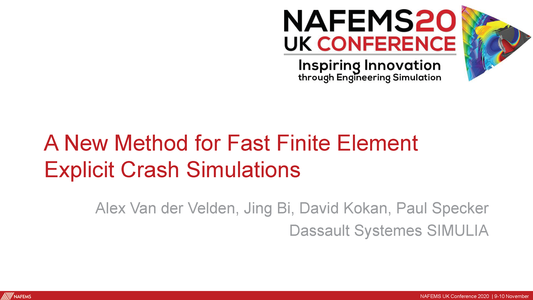
This presentation was held at the 2020 NAFEMS UK Conference "Inspiring Innovation through Engineering Simulation". The conference covered topics ranging from traditional FEA and CFD, to new and emerging areas including artificial intelligence, machine learning and EDA.
Resource AbstractToday, automotive crash finite element simulations are one or two orders of magnitude slower than stiffness, NVH and ride & handling simulations. Experimental validation studies show that fine meshes around 2mm are required to capture the metal folding behaviour with reasonable accuracy (10%). In addition, the stable time increment for explicit crash simulations is extremely short due to the small mesh sizes and the very high metal speed of sound. Therefore, a reasonably accurate full car crash simulation with 10 million elements will take more than three hours of simulation time, even when hundreds of cores are applied.
We present a new method to accelerate finite element explicit crash simulations by one or two orders of magnitude in order to enable multi-disciplinary concept and optimization studies. Since the collapse of thin walled structures is very dependent on local bending, torsion and compression conditions, it is critical that we represent the geometry with enough geometric refinement to support the concept design and with enough accuracy to match fine simulations to within about 15%. Our hypothesis was that the discretization error could be corrected out a priori for each mesh element on the basis of mesh geometry and material properties. For this purpose, we created a very large design of experiment study, which varied mesh geometry properties, material properties, material correction factors, component geometry characteristics and loading conditions. As outputs of the study we compared reaction forces, deformations and absorbed energy. We then captured the space with a machine learning interpolant and reduced the dimensional space to material stress-strain correction conditions whereby the outputs of the fine mesh matched the coarser mesh.
The material correction factors could then be applied a priori to complex components such as automotive S-beams and conceptual frontal structures without the need for iterative fine mesh calibrations.
To illustrate the power of this approach, we applied it to the design of 20 components of a simple conceptual automotive structure in a 55 km/h frontal crash scenario. The purpose of the design optimization is to reduce the driver accelerations to 35 g and limit the structural deformations to 550 mm. Such a structure is hard to optimize because changes in a single component can change the collapse sequence of the beams, thus creating a bifurcation in the crash response. We achieved good results in just 10 Adaptive DOE iterations, each iteration submitting all 50 frontal crash simulations in parallel, each simulation on multiple cores. The parallel nature of ADOE decreased the optimization time by a factor of 20, whereas the corrected coarse mesh simulation reduced the simulation time by another factor of 30, bringing the frontal crash optimization process from a month to less than an hour on the 3DEXPERIENCE platform.