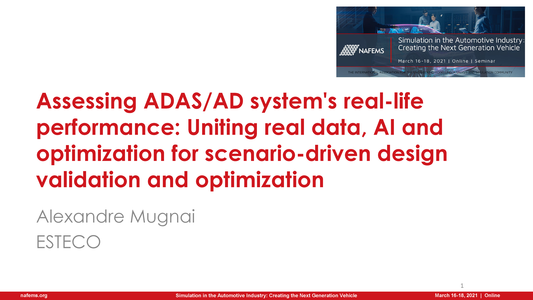
This presentation was made at the NAFEMS Americas Conference "Simulation in the Automotive Industry: Creating the Next Generation Vehicle" held on the 16-18 March 2021.
The automotive engineering community is now confronting the largest technology transformation since its inception. The demand to manufacture cleaner, safer, and smarter vehicles, along with providing an overall enhanced driving and ride experience, has never been higher. As a result, engineering teams must discover, evaluate, and successfully implement leading-edge technology and methods to produce reliable, effective results.
Hence, the challenges for automotive engineers are enormous and require a significant increase in the upfront use of numerical simulation capabilities, methods, and processes such that they’re able to efficiently design, manufacture and deliver these innovative technologies to market in greater speeds than ever before.
Resource AbstractADAS combines a number of components, including sensors (hardware and software processing), the algorithm fusing the data coming from multiple sensors, the algorithm deciding to act upon those inputs (braking, steering, accelerating), and finally, the actuators that will be implementing the decision. The Autonomous Driving cars shall and will work on the same principles of sensing, perception, decision and control.
For ADAS systems (level-1 and level-2 type) [refer to SAE levels], car manufacturers have been validating the algorithms mainly through physical testing - driving vehicles around and ensuring that a limited number of false positives would appear and software fixes will be done through traditional V loop of development and verification. However, with the increase in number and complexity of these functions, and the desire to move towards level-3/4 and level-5 autonomy, a considerable amount of testing becomes necessary for validation purposes, i.e., for certification purposes.
In total, at least 5 billion miles of driving would be required for proving with 95% confidence that the autonomous vehicle failure rate is lower than the human driver failure rate, with it being 20% better than the human fatality rate [refer to the RAND report].
The study presented here proposes a method that is timely and financially viable to validate and possibly certify an AD vehicle with extensive usage of simulations. Still to ensure simulation usage is optimized, the starting point is driving data out of which most relevant scenarios are identified. To achieve this objective, an Extreme Value Theory (EVT) algorithm has been created to distinguish critical use cases from rare use cases. This is also combined with an Inverse Reinforcement Learning (IRL) algorithm to have a predictive actor model which brings more realism in the actor behavior when used in a simulation environment.