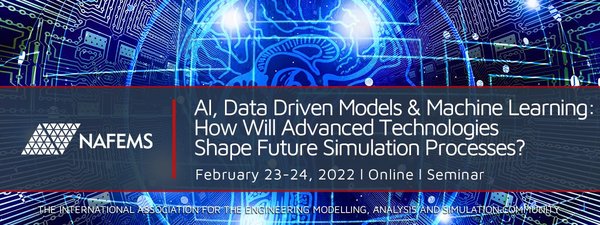
Photorealistic Synthetic Data Generation For AI-Based Feature Development
AI feature development for automated driving applications is heavily reliant on large
quantities of diverse data. Generative Adversarial Networks (GANs) are now widely used for
photo-realistic image synthesis. However, in applications where a simulated image needs to be
translated into a realistic image (sim-to-real), GANs trained on unpaired data from the two
domains are susceptible to failure in semantic content retention as the image is translated from
one domain to the other. This failure mode is more pronounced in cases where the real data lacks
content diversity, resulting in a content mismatch between the two domains - a situation often
encountered in real-world deployment. This presentation will discuss the role of the
discriminator's receptive field in GANs for unsupervised image-to-image translation with
mismatched data, and study its effect on semantic content retention. The presentation will also
show how targeted synthetic data augmentation - combining the benefits of gaming engine
simulations and sim-to-real GANs - can help fill gaps in static datasets for vision tasks such as
parking slot detection, lane detection and monocular depth estimation. Prior knowledge in
computer vision and deep learning will be helpful in getting the most out of this session.