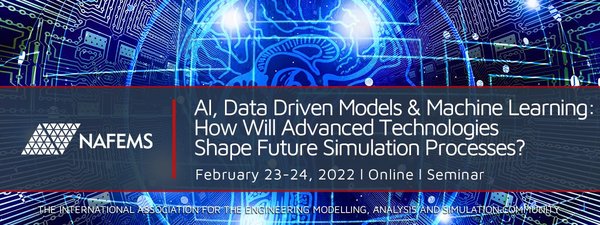
AI-Driven Learning of the Science and Engineering of Materials from Simulations
The increasing availability of data from the first three paradigms of science (experiments, theory, and simulations), along with advances in artificial intelligence and machine learning (AI/ML) techniques has offered unprecedented opportunities for data-driven science and discovery, which is the fourth paradigm of science. Within the arena of AI/ML, deep learning (DL) has emerged as a transformative technology in recent years with its ability to effectively work on raw big data, bypassing the (otherwise crucial) manual feature engineering step traditionally required for building accurate ML models, thus enabling numerous real-world applications, such as autonomous driving. In this talk, I will present some of the ongoing AI/ML/DL research in our group to learn the forward and inverse processing-structure-property-performance (PSPP) relationships in material systems using data from a variety of simulations -- such as density functional theory (DFT), finite element method (FEM), discrete dislocation dynamics (DDD), and rigorous coupled wave analysis (RCWA). We will also briefly discuss how AI models trained on simulations can be adapted for experimental data.