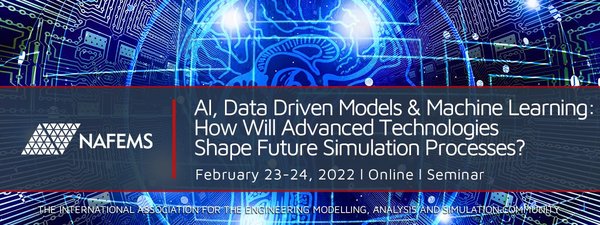
Hybrid Approach Combining Machine Learning and Meta-Modeling to Predict Material Behavior
In today’s highly competitive industrial landscape, we witness a pervasive economic pressure to constantly innovate and bring forward novel solutions at a faster rate.
In the field of materials and ICME, there is a strong interconnection between manufacturing processes, environmental conditions and the underlying microstructure that drives the part performance. Understanding these connections would require exhaustive testing and trial-and-error, which are set against the current pressure to rapidly innovate and shrink development times.
To this point, physical testing covering a broad spectrum of environmental scenarios and material conditions (temperature, strain rates, fiber content, additives, etc.) can be dauntingly large and impractical to cover exhaustively, leaving material suppliers and other OEMs to reluctantly accept large “voids” in the design space.
As such, we propose a hybrid workflow based on data analytics, machine learning and meta-modeling in order to build and predict highly reliable virtual results for a broad spectrum of temperatures, strain rates, humidity contents, material types, etc. These results can also be used to construct material models able to predict part performance under given test conditions with requisite accuracy.