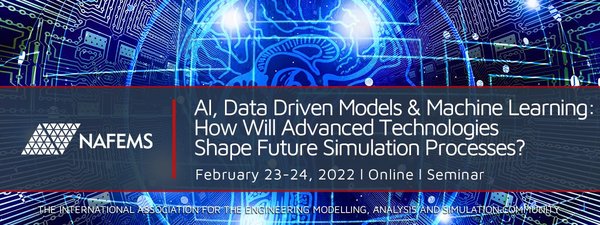
Neural Network Approaches for Plastic Material Modeling
Neural network approaches have been widely applied to constitutive modeling of elastoplastic materials, due to their ability to calibrate a large number of parameters and to approximate arbitrary mathematical equations. Some neural network approaches (e.g., recurrent neural network) can also be used to approximate history-dependent behavior. Given that material model calibration usually requires engineering expertise, neural network approaches offer an intriguing alternative. In this work, different neural network and hybrid approaches are utilized to model isotropic and anisotropic plastic materials. The feasibility to implement such methods into finite element analysis is evaluated. Focus is placed on adapting the existing methods to different material behavior and structural problems. Benchmark results show that the present models and methods can adequately predict different plasticity behavior.