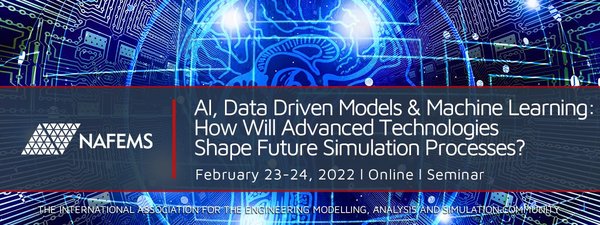
Accelerating Manufacturability Processes Using PINNs
Design optimization and identifying critical regions in a design fast is one of the primary objectives for the manufacturability of a new product. Each iteration using classical CAE methods can come with an enormous effort in time and cost. One major obstacle of using classic design optimization algorithms are speed and lack of important input and output information for critical applications.
The traditional CAE approach often forces engineers to run multiple parametric studies for new designs which not only creates huge data lakes but also makes this process very costly and tedious especially because the simulation must be re-run each time an engineer makes a change.
Surrogate models leveraged by Monolith AI can help overcome on-premise hardware restrictions and exploit raw data using them for training and getting new key insights from seemingly irrelevant old data lakes. This gives users an easy to interpret output such as the manufacturability of a component by learning from complex input data and mapping them to surface fields in an unparalleled fast manner and fewer simulations that need to be run in the long run. This patent-pending approach works on all types of meshes and has proven to be very efficient when varying the geometry and mapping tested conditions to geometries which have not be seen in training.