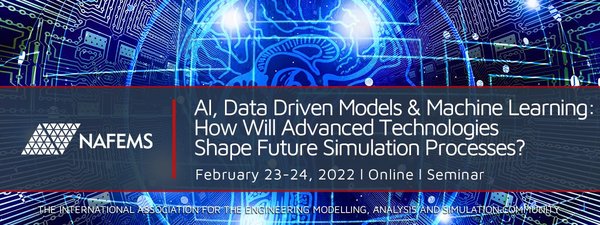
Critical Asset Predictive Maintenance with Data-Driven and Physics-Based Simulation Software
Unplanned downtime is one of the largest sources of lost production in chemical manufacturing processes. The average unplanned downtime for a mid-sized chemical plant costs approximately $5 million per year and 40% of the maintenance was unnecessary. Predictive maintenance (PdM) has made significant strides in recent years with advances in computational capabilities of data science, machine learning, and physics modeling. PdM now represents the strongest solution to this persistent manufacturing issue, but many manufacturers are still struggling to successfully implement these solutions. Successful IT-OT system integration is one of the challenges. Depending on how that is handled, is largely based on thought put into and identification of experienced deployment agents. After successful integration, the next problem is amount and quality of data. To provide accurate prognostics, you often need large amounts of specific data about when faults happen or run-to-failure information. A possible solution is to move away from purely using machine learning based solutions and instead use a combination of advanced data-driven machine learning and physics-based algorithms to monitor critical assets for anomalies and prognostics of remaining useful life (RUL). Use of subject matter expertise to fill in the gaps in the data, identify good versus bad data, and to create models and algorithms that ensure that physics is correctly accounted for is very important.
In this presentation, we will talk about the science behind these models, how they can be made configurable and easy to deploy, and calibrated on the fly or with OEM information. We will also include a few examples of anomaly detection and prognostics models based on physics-based and machine learning models and how they are applied to real world situations. Some of the common assets-valves, seals, heat exchangers, and pumps-found in the chemical industry will be covered. We will also present business use cases that resulted in up to a 6-month lead time with 95% accuracy of critical failure on certain assets.